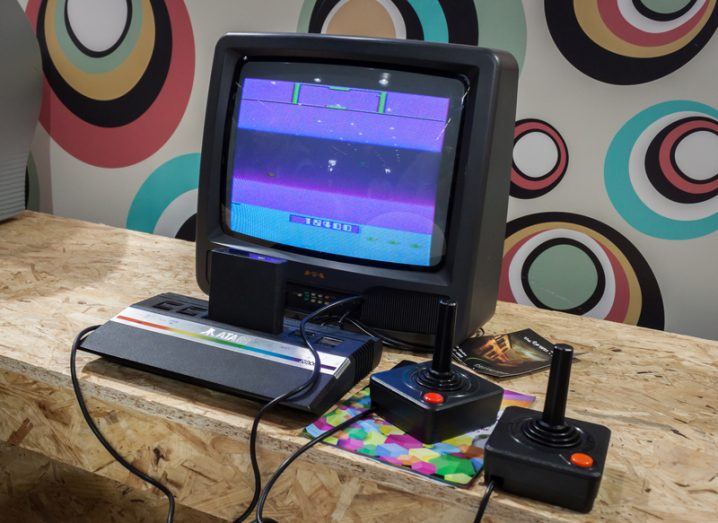
Atari video game console. Image: Tinxi/Shutterstock
Google’s DeepMind AI has proven itself as an accomplished video game player, but its latest achievement could be the first glimpse of true memory.
Under Google, the artificial intelligence (AI) research company DeepMind has greatly expanded its capabilities, particularly when it comes to games, both virtual and physical.
Its AlphaGo project – whereby its AI was pitted against some of the best Go players in the world – proved that it could not only beat the best player in the world, but it could also trick human players into thinking they were playing a human as well.
Similarly, beginning in 2014, DeepMind trained its machine learning systems to play a number of classic Atari video games, showing it had the ability to outperform human players.
Now, according to Wired, DeepMind has revealed that its gaming prowess is now so advanced, that it is capable of beating a video game based on things it has learned from playing other video games.
This would indicate that the new algorithm defining the latest version of its AI – called elastic weight consolidation (EWC) – has given it the human ability of memory.
Using the previous model, the AI could indeed be taught to play a video game. However, each time, a separate neural network had to be created, meaning it wasn’t really remembering anything.
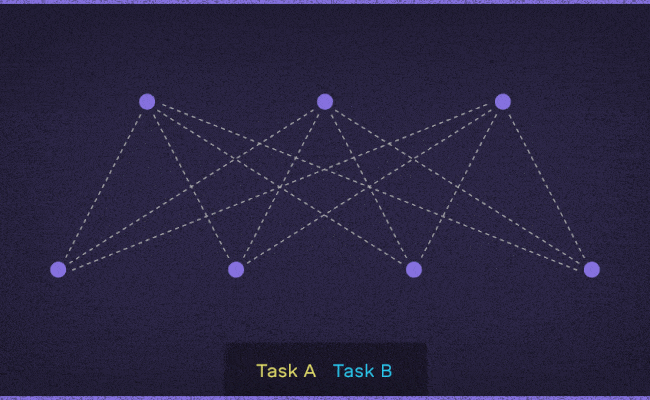
Illustration of the learning process for two tasks using EWC. Image: DeepMind
Remembers, but very slowly
In the latest experiment, DeepMind and a team from Imperial College London developed the algorithm with the intention of making its neural network use supervised learning to remember sequences, as explained in the journal Proceedings of the National Academy of Sciences.
Lead author of the paper, James Kirkpatrick, explained that the EWC algorithm takes information from the way it learned to beat previous games, and then slowly transitions some of these memories into a new game.
So slowly, in fact, that the AI would play each Atari game 20m times before moving on to the next one.
This added complexity makes it no match for one of the neural networks programmed to play just one game.
“At the moment, we have demonstrated sequential learning but we haven’t proved it is an improvement on the efficiency of learning,” Kirkpatrick said.
“Our next steps are going to try and leverage sequential learning to try and improve on real-world learning.”
Atari video game console. Image: Tinxi/Shutterstock