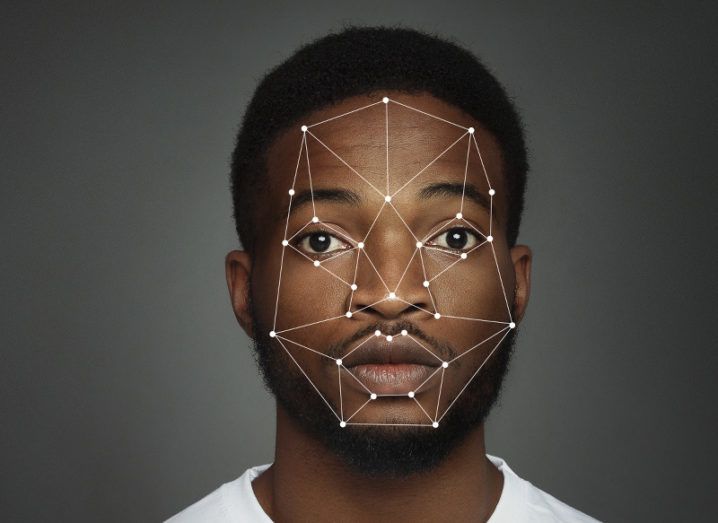
Image: © Prostock-studio/Stock.adobe.com
In showing the data behind the machine, ImageNet Roulette has revealed how human racism and bias can be become embedded in robotic systems.
You may have seen screenshots from ImageNet Roulette circulating on social platforms as the latest viral selfie-analyser took off.
ImageNet Roulette works a bit differently to the FaceApps, arty doppelgangers, age guessers and more facial recognition apps that have come before it. It somewhat reverse engineers the process to give you a window into the data that humans fed into the neural network to begin with.
‘We want to shed light on what happens when technical systems are trained on problematic training data’
– IMAGENET ROULETTE
ImageNet Roulette uses data from ImageNet, a dataset released in 2009 by researchers at Stanford and Princeton who used Amazon’s Mechanical Turk to crowdsource the labour required to classify and label its 14m images. This expansive dataset has since seen common use in the training of algorithms for object recognition and has been cited in hundreds of scientific publications.
ImageNet Roulette scans an uploaded image for faces and then finds a fitting classification among the 2,833 ‘person’ categories in the dataset’s taxonomy. It all seemed like a bit of harmless fun, with some people realising their face was labelled that of a pilot or newsreader, but then technology reporter Julia Carrie Wong took ImageNet Roulette for a spin.
tfw when you get a press release about an AI photo thing that you've seen lots of other tech reporters having fun with but then it's actually not that fun pic.twitter.com/NMZNxlGNZW
— Julia Carrie Wong (@juliacarriew) September 17, 2019
Wong, who is biracial, received the offensive slurs ‘gook’ and ‘slant-eye’ as labels for her image. “People usually assume that I’m any ethnicity but Chinese. Having a piece of technology affirm my identity with a racist and dehumanising slur is strange,” she wrote of her experience.
Offensive by design
Wong and others who received offensive and questionable results from this tool have highlighted the ease with which prejudice, bias and outright racism can become embedded in robotic systems.
In fact, revealing what lies beneath the algorithm is the purpose of the project from AI researcher Kate Crawford and artist Trevor Paglen.
The tool’s website explains that the dataset contains a number of “problematic, offensive and bizarre categories” derived from WordNet, a database of word classifications developed at Princeton University in the 1980s. This includes racist and misogynistic language and so, offensive results cropping up is no glitch.
“We want to shed light on what happens when technical systems are trained on problematic training data. AI classifications of people are rarely made visible to the people being classified. ImageNet Roulette provides a glimpse into that process – and to show the ways things can go wrong,” the website claims.
Whoa, ImageNet Roulette went… nuts. The servers are barely standing. Good to see this simple interface generate an international critical discussion about the race & gender politics of classification in AI, and how training data can harm. More here: https://t.co/m0Pi5GOmgv pic.twitter.com/0HgYsTewbx
— Kate Crawford (@katecrawford) September 18, 2019
Database update
Following the surge in popularity of ImageNet Roulette, an update was posted to the ImageNet website yesterday (17 September). “Over the past year, we have been conducting a research project to systematically identify and remedy fairness issues that resulted from the data collection process in the people subtree of ImageNet,” the researchers, including Stanford’s Prof Fei-Fei Lee, wrote. Specifically, they identified issues such as the inclusion of offensive terms and a lack of diversity in the source images.
Among the 2,382 people subcategories, the researchers have decided to remove 1,593 that have been deemed ‘unsafe’ and ‘sensitive’. This will result in the loss of more than 600,000 images from the database but will leave more than half a million images of people for neural networks to work with.
The researchers’ update further ponders the ethics of classifying people even with ‘safe’ subcategories and explains a solution in progress to rebalance the demographics in the dataset.