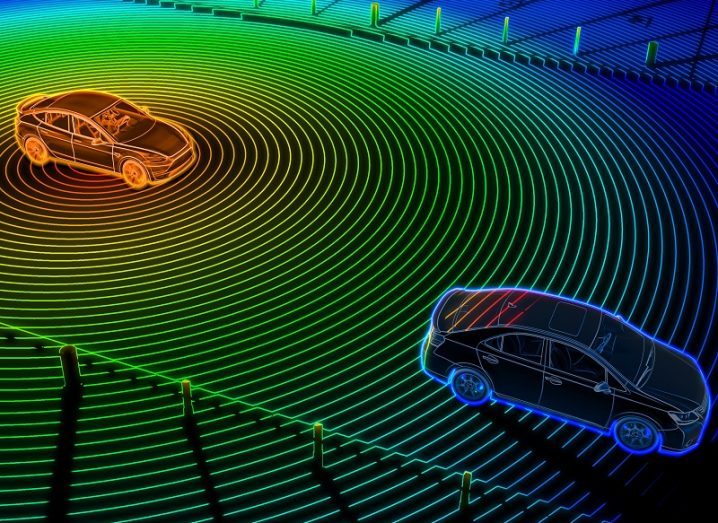
Image: © temp-64GTX/Stock.adobe.com
Mohammad Musa, co-founder and CEO of the start-up Deepen AI, gives a unique insight into the future of autonomous vehicle sensors.
The recent announcement by Waymo that it would start selling low-priced LiDAR sensors should have been a great signal to the broader autonomous vehicle (AV) industry. Until now, a handful of the largest companies have held significant advantages in AV development. One of them marketing its proprietary sensor tech is a sign that the industry is developing into a rational ecosystem, where at least safety-related tech is shared (for a price, of course).
Unfortunately, Waymo says it will not sell the tech to others building autonomous vehicles, so the signal is not quite as strong as I’d have liked. Nevertheless, selling the tech to adjacent industries means the cat is out of the bag.
LiDAR sensors will be much more broadly available at an affordable price, and this will inevitably – and I predict fairly quickly – bring cheaper LiDAR sensors to the AV world. That will help all of us in the industry move to the multisensor model we so need.
What’s so important about multisensor systems? It’s simple: nothing is more critical to the mass deployment of Level 4 and Level 5 autonomous vehicles than refining the sensory inputs AVs use to navigate. (As a rule of thumb, you can think of Level 4 as autonomous vehicles at least as safe as human drivers in most situations, and Level 5 as AVs safe in all situations.)
Camera technology – the most widely used sensor tech today – has its limitations, as it only offers the ability to perceive the environment in two dimensions with a wide field of view. Some systems augment that with radar, but using input data from just the camera and radar isn’t even enough.
Achieving Level 4 and Level 5 AVs will require using not just one or two, but rather multiple sensory inputs. Each of these complement each other well and nothing complements camera plus radar better than LiDAR.
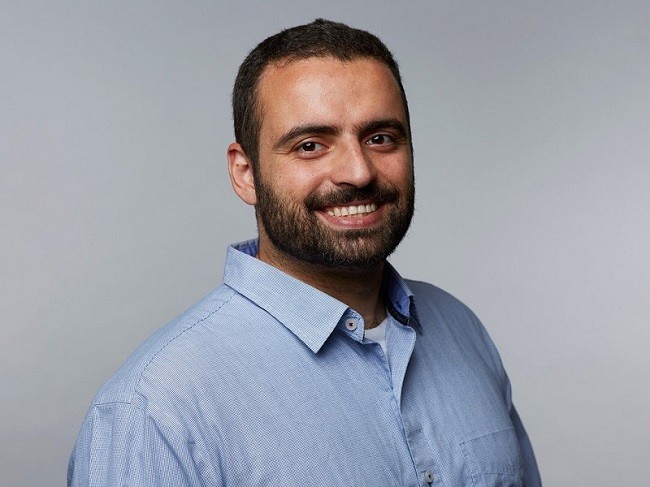
Mohammad Musa, CEO and co-founder, Deepen AI. Image: Deepen AI
Opening up the senses
So, what’s it like to train an AV with just one or two sensory inputs? Imagine you were asked to bake a cake. With a good recipe and the right ingredients, the task would probably be doable, even if you’ve never baked a cake before. Now imagine you were given this task, but your sense of taste was taken from you. Or perhaps you were blindfolded. After enough attempts, you might finally get it right, but you wouldn’t be able to bake that cake consistently unless you had use of all possible sensory information.
It’s no different with AVs. Impressive progress has been made in developing radar and camera data, but other sensory mechanisms – such as sonar, LiDAR, infrared and even acoustics – have by and large been underutilised by the AV industry.
Most would agree that multisensor data can provide far more vivid and complete information than those built from just one or two sensory inputs. This is fairly intuitive; a greater quantity of quality data enables better judgement and more informed decisions in almost every circumstance imaginable.
And yet, a further implication of multisensor data is routinely overlooked: that it’s the only way to reach an adequate level of safety for the mass deployment of AVs, not to mention the key milestones on the way there.
Along with having a more complete sensory map when everything is running correctly, when one or more inputs are disrupted (perhaps due to a mechanical error or the weather), the other inputs are able to act as a sort of failsafe. If the technology behind each sensory input develops properly, no mechanical failure or outside factor should ever make the AV a safety hazard, neither for the passengers inside the vehicle nor anyone or anything in its path.
Making sense of LiDAR
While some of the less-developed sensory inputs (sonar, infrared, acoustic) are familiar to most, LiDAR is generally the least understood – and it may be the most capable of all of these. LiDAR refers to motion and object detection in 3D through the use of lasers. Not only can it provide incredibly precise information on even the smallest objects within a radius of about 60 metres, but it is constantly able to observe its surroundings in 360 degrees.
LiDAR provides much more granular information than radar – not that it’s meant to replace radar. Rather, AV sensory maps are most effective when the two technologies work in harmony with one another.
LiDAR is not, of course, without its drawbacks. Snow and rain can disrupt LiDAR readings, which is why they’re most effective as a complement to other sensory inputs. LiDAR systems that use lasers with short wavelengths are far less effective than those that use longer ones.
LiDAR is also aesthetically limited. The design of LiDAR devices is so typically bulky and unusual that it’s one of the reasons Elon Musk said he’ll never put them on his cars. “Perhaps I am wrong,” he said, “and I will look like a fool.”
A new type of LiDAR that’s not yet widely available has added a new wrinkle to the sensor landscape: FMCW LiDAR. Rather than pulsed laser technologies with traditional LiDAR sensors, FMCW LiDAR is based on frequency modulation.
Think of it this way: traditional pulsing LiDAR is like AM radio, whereas FMCW LiDAR is akin to FM radio. These sensors offer greatly improved interference rejection and long-range detection. They also provide a measure of the velocity of every point measured. Many experts see these sensors as the future of automotive LiDAR technology.
“The addition of a velocity reading to the point-cloud data provides an enormous boost to just about every task in the perception stack: object segmentation and tracking, object classification, ego-motion estimation and compensation, localisation, and mapping, just to name a few,” said Jim Curry, vice-president of analytics at Blackmore, a leading provider of FMCW LiDAR sensors.
Perhaps the most consequential problem most manufacturers face when it comes to LiDAR is that they simply don’t have access to enough data to test and develop it effectively. Systems need to be trained on millions and millions of annotated LiDAR points in order to have data that’s in any way reliable.
So far, no workaround has been adopted on an industry-wide basis. (Waymo is the lone exception; the company can lean on useful LiDAR insights thanks to a suite of software tools it developed in-house for LiDAR and multisensor data.) Plus, changes in the LiDAR industry happen rapidly, further underlining the industry’s need for good annotation tools related to this technology.
Ultimately, using LiDAR along with other sensory inputs – radar, video, infrared, acoustics – is the best way the AV industry can hit a critical yet often overlooked milestone: more sophisticated driver assistance technology. Even as other sensory technology develops, there simply isn’t a path for achieving sufficient AV safety and Level 4 driverless technology besides training multiple sensory inputs, including LiDAR, together.
Crawl before you walk
It’s probably disappointing for many to realise that a future in which highways are driven on mostly by AVs is probably a bit further away than what’s been promised. But the realism is good: we now have a clearer understanding of the milestones that need to be hit on the way to full AV industrialisation.
The clearest milestone ahead of us right now is the development of driver assistance technology that’s much more reliable than what most manufacturers have today. Despite the data challenges most manufacturers face, the importance of developing sensory maps that involve multiple inputs, including LiDAR, cannot be understated.
With more reliable and accessible data from all sensory inputs, the journey to Level 5 AVs – not to mention the major milestones along the way – becomes far less daunting.
Mohammad Musa co-founded Deepen AI in 2017 to solve critical bottlenecks preventing faster adoption of autonomy and robotics products. He was a product strategy manager for Google Apps, now part of the Google Cloud platform. Before Google, he worked as a software engineer at Havok (acquired by Intel), Emergent Game Technology (acquired by Gamebase) and Sonics (acquired by Facebook).