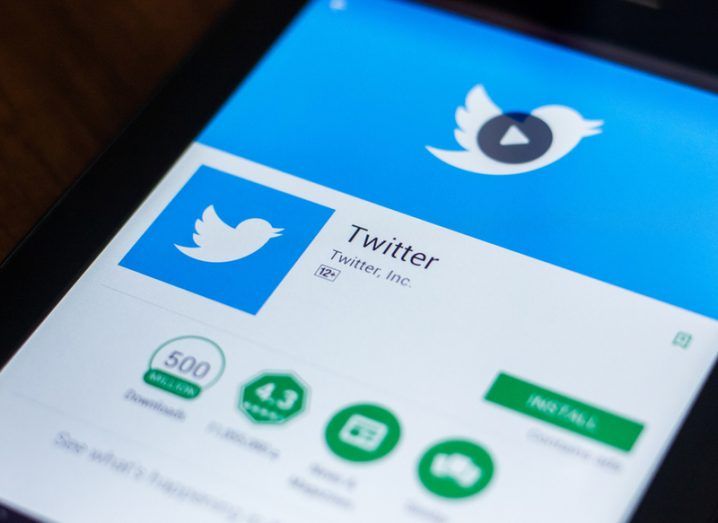
Image: Sharaf Maksumov/Shutterstock
Botnets can thrive on Twitter, but how do they avoid detection?
Twitter botnets have been an area of interest for security experts and the average user of the platform for some time now. Whether it’s spreading spam, advertising cryptocurrency scams or potentially influencing the democratic process, bots have become a key research area for infosec professionals.
A report published yesterday (6 August) by Duo Security researchers examined just how bots and botnets are created, as well as how they use clever tactics to evade detection. Written and researched by Olabode Enise, data scientist at Duo, and the company’s principal R&D engineer Jordan Wright, the Don’t @ Me report is an exploration of hunting these bots at scale.
The researchers first had to build a dataset by fetching public Twitter profiles. They then fortified this by gathering tweets sent from these users. In doing so, they were able to note links between certain accounts. “For example, once a bot is identified, the bot’s social network information can be gathered to find similar connected accounts, resulting in a network that could be a potential botnet,” the report explained.
To identify a bot, the researchers examined three areas.
Account attributes
These are factors that include the number of tweets or likes, length of time an account has been active, or screen name. Researchers noted that bots often had numerous digits as well as varying degrees of entropy in their usernames.
They wrote: “We observed cases where accounts had no activity other than liking many tweets in a short period of time, raising the possibility that the account may be an amplification bot designed to artificially inflate the popularity of tweets.”
Content
Attributes analysed included the appearance of URLs in tweets, which can often be an indicator of a user who actively shares links or a potentially malicious bot.
Metadata
Metadata can be the most revealing, as the authors explained: “A great example of this is time. For example, the average Twitter user will likely only tweet during certain hours of the day, whereas bots are able to tweet throughout the entire day.
“This same analysis can be performed on other tweet relationships, such as how quickly consecutive tweets are posted, how quickly replies are generated, or how quickly a tweet is retweeted.”
How do you wrangle bots at scale?
The researchers used machine learning (ML) algorithms to locate lots of bots quickly. They used features such as rate of tweets liked compared to the account’s age, number of numeric characters in a username and others to train the ML models.
They noted the study did have some limitations in terms of being unable to consider the entire timeline of an account and behaviour changes seen here.
What are cryptocurrency bots doing to evade detection?
- Using screen names that are typos of a spoofed account’s screen name
- Performing minor editing on the profile picture to avoid image detection
- Using Unicode characters in tweets instead of traditional ASCII characters
- Adding various white spaces between words or punctuation
- Transitioning to spoofing celebrities and high-profile Twitter accounts in addition to cryptocurrency accounts
Fighting Twitter botnets
The researchers said: “During this research and in our conversations with Twitter when sharing our analysis, an area that emerged as being important to any future research was the difference between the view of Twitter built through its API and what users see in the user interface (UI).
“According to the company, Twitter is actively working to hide malicious content from being visible in areas like search and conversations, though the content can still be visible via the API.
“Twitter cites that ‘less than 5pc’ of accounts are spam related. Differences between data exposed via the UI and API, and the security ramifications of these differences, is an area we are excited to explore further in future work.”
Wright told Siliconrepublic.com: “By open-sourcing our code and techniques detailing the entire process of finding bots, we’re excited to enable the community of incredibly talented researchers to build on our work and continue finding new and innovative ways to tackle bots and larger botnets.
“In the future, we anticipate researchers can use the work we’re publishing to ‘get more eyes’ on the issue of detecting bots, resulting in even better detection and quicker response.”