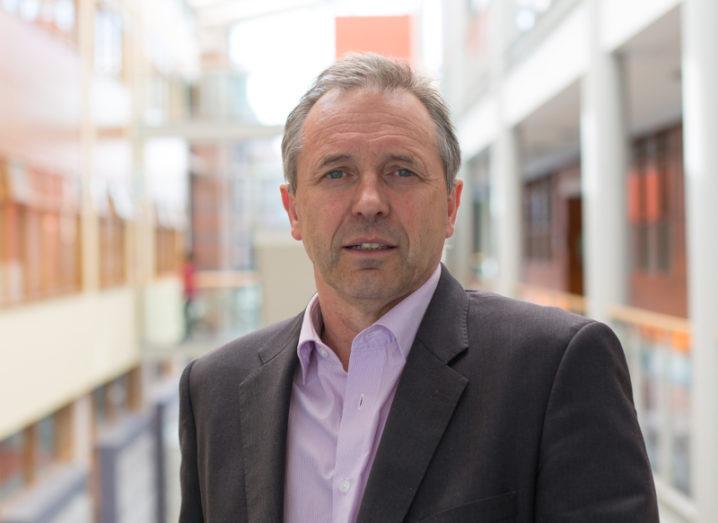
Prof Alan Smeaton. Image: DCU
Prof Alan Smeaton, director of the Insight Centre for Data Analytics, discusses why good data science – and good science overall – requires open sharing of information.
Fake news is shaking our faith in information. Unfortunately, public loss of trust in news sources doesn’t just impact on irresponsible media outlets. It undermines trust in all information sources.
Coupled with an emerging virulent anti-intellectual strain in public debate, it’s getting harder for news based on data analytics or any form of machine learning to cut through the noise. The emergence of easy self-publishing through social media outlets like Twitter and Facebook – which many people now use as their primary source of news – means that we are flooded with information we are ill-equipped to process. In addition, most people lack the skills and knowledge to make value judgements as to the truth or otherwise of the news they read.
When it comes to news about peer-reviewed science in general, we have a similar problem. Imperfect as it is, the scientific method is more rigorous than any other information dissemination model. It is founded on the principle that other scientists must be able to replicate the work of the published scientist, use their data and get the same results, and then extend that work with new ideas.
In the age of big data, this is particularly important, because data is not blind. How it’s collected, when, where and what for – all of these factors have a bearing on the headline findings that can then turn into news.
Good data science – and responsible science in general – is about making your data, your tools and your techniques openly available to others. This is the current vogue in publicly funded science. Not just to fact check our claims, but also to allow newly developed techniques to be tried on others’ data.
Statistics and correlation
We’re very good in science, generally, at sharing the outputs of our work, and we now provide open and easy access to most of our own research publications. Our national and European research funding agencies mandate that all our researchers use open access digital libraries, such as those that our third-level institutions now provide.
A case in point is an article published in The Lancet recently, which reported a study of 243,611 incident cases of dementia in Canada, concluding that the risk of contracting Alzheimer’s disease could be increased by living close to busy roads. This was picked up by most of the major news outlets globally – including many reports in Irish news – and went viral. A similar scare can be seen around the recent news item connecting burnt toast with cancer, for which there is no current scientific basis.
While there may indeed be a link between Alzheimer’s and living proximity to road traffic, there are quixotic occurrences in all data collections. The inexplicable correlation between chocolate consumption and serial homicide in different countries is a classic example. It is a statistical fact that there are more serial homicides and more chocolate consumption, per capita, in a country like Norway than in Italy or Japan. There is no rational explanation for this correlation, but it’s a statistical fact.
‘Democratisation of research data is an important goal for the scientific community’
Good data science is about not just publishing and sharing the results of research. It should also be about sharing the data. It may indeed be true that exposure to air pollutants and diesel exhaust caused by living close to a busy road does induce oxidative stress. It may also be true that exposure to more road noise impairs cognitive abilities and these in turn may be triggers for Alzheimer’s.
Yet there may be other factors that the authors missed. For example, employment status, local precipitation where rainfall can ‘clean’ the air of particulate pollution, housing or socio-economic status – all these and more may also play roles. This invites us to look at such factors and see beyond the immediate, apparent correlation.
Secondary analysis
Without the raw data, however, other data scientists like myself cannot pursue these avenues. The Canadian study used individual-level health records and so the dataset is not publicly available, even in anonymised form.
As a data scientist keen to use artificial intelligence, machine learning and other data science tools for secondary analysis, I cannot examine this analysis further as it would require moving my research to Ontario, Canada, from where it originated.
The current norm for sharing and re-using data like this is not yet at the sweet spot that balances facilitating research for public good and protecting the privacy and ownership of personal or clinical data.
This issue is very topical and the New England Journal of Medicine published several articles on this last year, looking at the balance between fairness to the original researchers who gathered and cleaned the data, value for the taxpayer who funded the data collection research in the first place, payback for the individuals who may have donated the data, and the population at large who could benefit from secondary processing of the data.
Democratisation of research data is an important goal for the scientific community if we are to continue to build public trust in the information we provide. This is a critical public service in an age when no one knows who to believe anymore.
Prof Alan Smeaton is a professor of computing at Dublin City University (DCU) and director of the Insight Centre for Data Analytics at DCU. He has published over 300 book chapters, and journal and refereed conference papers, as well as dozens of other presentations, seminars and posters.