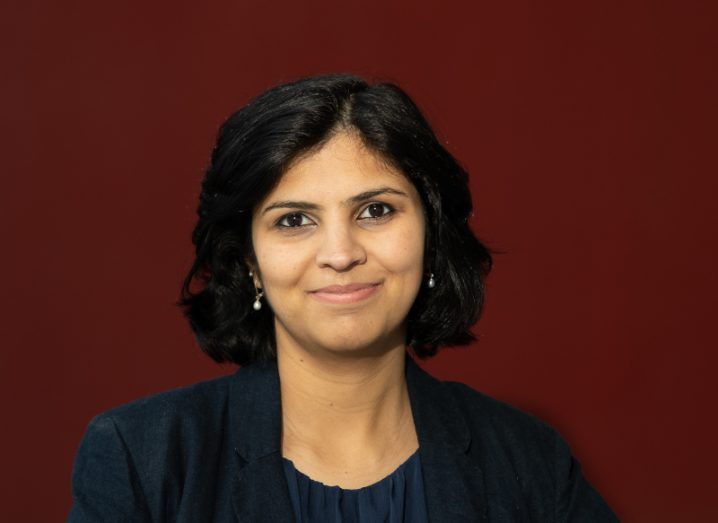
Pallavi Tiwari. Image: Matt Shiffler
Dr Pallavi Tiwari and her team at the Brain Image Computing Laboratory are using AI models to help move away from a one-size-fits-all approach to treating brain tumours.
As technology continues to advance, its use within the healthcare sector is becoming more and more prominent. From remote healthcare to 3D printing, there are so many applications of technology that can pave the way for personalised medicine and better healthcare.
One such application is the use of AI and machine learning in the treatment of brain tumours, something I spoke to Dr Pallavi Tiwari about.
Tiwari is an assistant professor of biomedical engineering and the director of the Brain Image Computing Laboratory at Case Western Reserve University in Ohio.
While she was always passionate about the field of STEM and engineering in particular, her interest in automation and data analysis only began during her sophomore year at college in India, where she worked on building a navigation system for the visually impaired, which won second place at a major tech festival for translational biomedical technology.
“This win came as a huge surprise and made me realise that my ideas could have real impact. My foray in technology development started from there and my interests were further reinforced during my master’s work at Rutgers University,” she said.
“At Rutgers, I got the opportunity to work in a growing lab with an outstanding mentor, who I have learned a lot from over the years. I worked on developing computational tools for prostate cancer grading.”
Tiwari then turned her attention towards developing prognostic and predictive computational tools for personalised treatments in brain tumours, which is where her current focus lies.
She is leading a team of researchers on multiple projects using AI and image informatics for prognosis and treatment evaluation in adult and pediatric brain tumours.
“We focus on using machine learning approaches to address questions for personalising treatment decisions such as who to treat, how to treat, did the treatment work? These are all critical, time-sensitive questions, the answers to which are often nebulous due to the lack of sufficient diagnostic information based on current clinical care.”
‘We exploit information from routine clinical MRI scans and do not require any additional expensive imaging examinations’
– PALLAVI TIWARI
Tiwari’s group focuses on glioblastoma, an aggressive brain cancer with an average survival time of between 12 and 18 months. While treatment for other cancers has changed over the years, glioblastoma treatment still requires chemoradiation, which does not suit every patient.
“Chemotherapy is a cytotoxic drug and has significant side effects. The question that we are asking is can we optimise treatment decisions for these patients so patients who are not suited for chemotherapy are not being subjected to this drug and perhaps may be more suited for other experimental treatments,” said Tiwari.
“We have recently also demonstrated that the tumour signatures at imaging and genomic scale, across men and women, are different. These findings could have significant implications in personalising gender-specific treatment decisions in brain tumour patients.”
A non-disruptive approach
This work is done at the Brain Image Computing Laboratory, which aims to develop neuroinformatic techniques using machine learning, statistical modelling and pattern recognition for applications in brain tumours and neurological disorders.
“A significant advantage of our machine learning approaches is that we exploit information from routine clinical MRI scans, so our approaches are clinical, non-disruptive and do not require any additional expensive imaging examinations,” she said.
“Additionally, our AI models are developed to characterise tumour heterogeneity specific to every patient and hence can be used to make personalised treatment decisions instead of choosing one-size-fit-all treatment strategies.”
Another project is developing machine learning approaches to tell the difference between benign side-effects of radiation and tumour recurrence.
Tiwari explained that because these two conditions mimic each other, a significant number of brain tumour patients may have to undergo unnecessary invasive surgery to identify the presence of a benign condition.
“Our methods are close to 90pc accurate in identifying benign radiation effects from tumour recurrence using clinical MRI scans. We are currently in the process of evaluating these approaches in the context of clinical trial data sets so they can be incorporated in clinical practice for decision making in the near future.”
While the use of AI and machine learning in healthcare can be revolutionary, it also requires buy-in from several stakeholders. However, Tiwari said people are starting to realise machine learning’s potential in clinical decision making.
“More and more technologies are getting regulatory approvals for clinical use. I am hopeful that this influx of machine learning approaches will start to have massive impact in the coming years as clinical decision support in assisting radiologists and oncologists make more informed decisions regarding personalised treatment decisions,” she said.
“The purpose of these techniques is not to replace the end users but to augment their decision process with complementary information that is perhaps not discernible via existing imaging approaches. Our group has been fortunate to work with some of the leading clinicians in the field who over the years have become the biggest proponents of our work and continue to be our cheerleaders in the clinical community.”
Don’t miss out on the knowledge you need to succeed. Sign up for the Daily Brief, Silicon Republic’s digest of need-to-know sci-tech news.