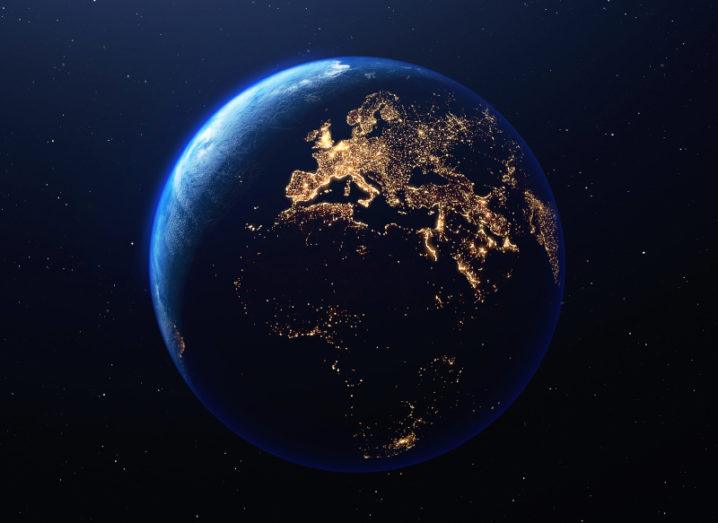
Image: © jim/Stock.adobe.com
Researchers in Ireland are looking to better facilitate the training of machine learning models with earth observation data.
The Irish Centre for High-End Computing (ICHEC) based at NUI Galway and Irish applied AI centre CeADAR have collaborated on a project to address the lack of standardisation in earth observation datasets.
The project was funded by the European Space Agency (ESA). The ESA’s Sentinel Satellite-1, Satellite-2 and Satellite-3 collectively produce an estimated 20 terabytes of data per day, and so are prime candidates when it comes to using AI to help with data analysis.
The Sentinel data includes C-band synthetic aperture radar imaging, which enables satellites to acquire imagery regardless of the weather. It also covers high-resolution optical imagery of agriculture, forests, land-use change, as well as sea surface topography, sea and land surface temperature, and ocean and land surface colour.
“The value of satellite data to projects which inform environmental policies, climate knowledge and mitigation strategies is unique,” Dr Jenny Hanafin, earth observation programme manager at ICHEC.
“However, until now there have been bottlenecks holding back the use of this data in [AI] applications.”
The project at ICHEC and CeADAR aims to improve the ability to share training data for scientific research and for the commercial and technical AI community, as well as lower the cost of sharing this data.
It is doing this by introducing new specifications and best-practice guidelines for creating datasets. By providing common specifications so that training datasets follow FAIR principles, data produced for one application will be made available for other users and uses.
These principles emphasise the capacity of computational systems to find, access, interoperate and reuse data with none or minimal human intervention, as humans rely on computational intervention to assess an ever-increasing amount data.
“This project set out to produce resources to support the training and development of machine learning models on EO [Earth observation] data,” Alastair McKinstry, environmental programme manager at ICHEC, said.
“The aim is to move towards implementing FAIR data principles for training data in EO, ensuring that datasets are properly documented and available to other users.
“Each dataset is a valuable resource … and facilitating the understanding and sharing of these data resources is the main goal. An additional goal is to make EO training datasets self-explanatory in order to expose challenging problems to a wider audience that does not have expert geospatial knowledge.”