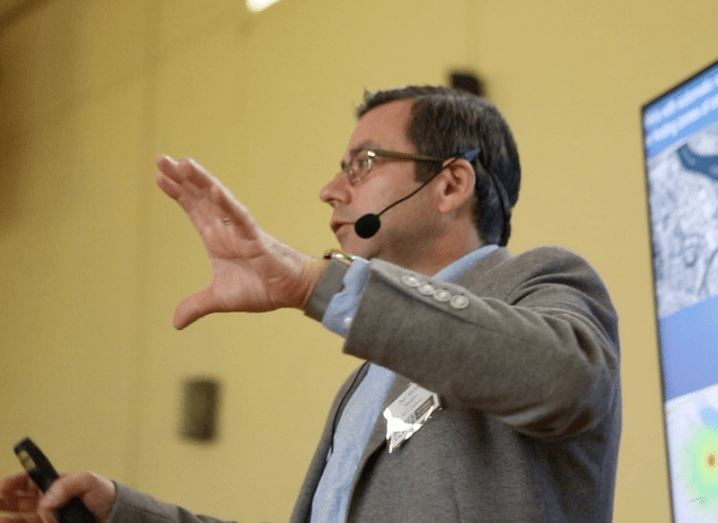
Prof Michael Madden, NUI Galway. Image: Luke Maxwell
Prof Michael Madden of NUI Galway coordinates the ROCSAFE project using AI, robots and drones to investigate hazardous crime scenes.
After graduating with a degree in mechanical engineering from NUI Galway, Prof Michael Madden began a PhD in machine learning. After turning to industry for a few years at a software company, Madden returned to NUI Galway as lecturer and set up its machine learning and data mining research group in 2001.
In early 2018, he was appointed as the established professor and chair of computer science at NUI Galway.
What inspired you to become a researcher?
From the age of about 11, I wanted to be an inventor as I had read books about famous inventors, though I was not clear on how to become one. When we first got a computer at home – a Sinclair Spectrum – I realised I could invent games and more by learning to program them.
Can you tell us about the research you’re currently working on?
With my research group, I work on new theoretical advances in machine learning (ML), including reinforcement learning, Bayesian learning, probabilistic reasoning, deep neural networks and other ML techniques. We seek to tackle problems in scientific and engineering domains including health and security.
To date, my research has led to 100 publications, four patents, 12 PhD graduates and a spin-out company. It has informed my teaching of ML and deep learning on our postgraduate programmes in data analytics and AI.
I am the coordinator of a project called ROCSAFE (Remotely Operated CBRN Scene Assessment and Forensic Examination) that is funded by the EU’s Horizon 2020 programme. The goal of ROCSAFE is to fundamentally improve how chemical, biological and radiation/nuclear (CBRN) incidents are assessed, and to protect the lives of crime scene investigators by reducing their need to enter dangerous scenes to gather evidence.
In your opinion, why is your research important?
The pace of ML research internationally has increased in recent years, driven by the convergence of a number of factors. These include new theoretical advances, availability of very large training data sets, open-source software frameworks, and new massively parallel computer architectures. As performance of ML systems improves, new opportunities are opening up for applications right across science, engineering and medicine.
What commercial applications do you foresee for your research?
In all of my research, we aim to solve practical real-world problems in science, engineering and health. My research on ML applied to analysis of chemical spectroscopy data has led to a spin-out company, Analyze IQ Limited, that commercialised the research results.
The ROCSAFE project has clear commercial applications in forensic science and first response, and five of the partner organisations are SMEs that will further commercialise specific research results.
What are some of the biggest challenges you face as a researcher in your field?
Current ML systems can come close to human-level performance, or even exceed human-level performance, in tasks that are carefully defined and constrained. These typically require very large quantities of labelled training data.
A key challenge for the field of ML in future years is to move from such narrowly defined tasks to broader modalities of learning, where machines can be less dependent on learning from labelled data. Instead, they could make more use of expert knowledge and transfer knowledge appropriately from one domain to another.
Are there any common misconceptions about this area of research?
There are some misconceptions about AI and ML because of all of the hype around these fields at present. Because we don’t fully understand human intelligence and learning, people often expect AI and ML to be somehow mystical or magical and can be disillusioned to discover it is underpinned by prosaic maths and programming.
At the same time, there are unrealistic fears about AI threats such as a ‘robot uprising’, while missing more practical threats of surveillance capitalism. I think these could be addressed by greater public communication and perhaps publicly available courses – possibly delivered via schools or clubs such as CoderDojo – about AI literacy.
What are some of the areas of research you’d like to see tackled in the years ahead?
While there have been exciting developments and applications, there is still much more to be done to ensure that systems that appear to work well in lab conditions transfer into the real world. Topics such as representation of human knowledge, handling uncertainty and guarding against bias are also important.
Are you a researcher with an interesting project to share? Let us know by emailing editorial@siliconrepublic.com with the subject line ‘Science Uncovered’.