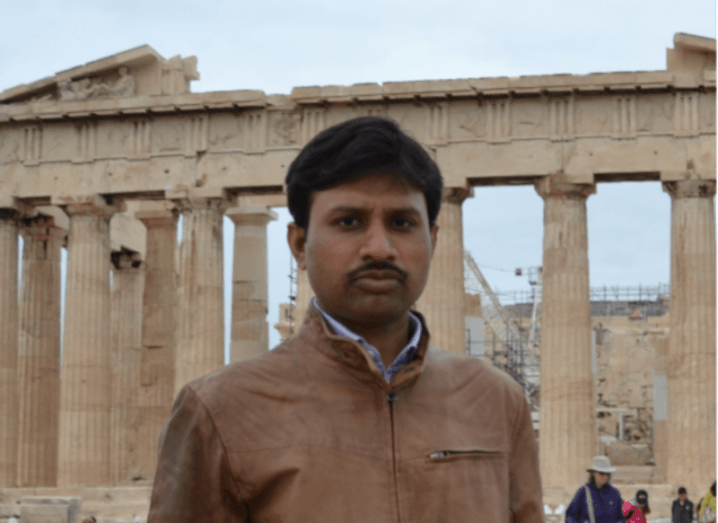
Image: Dr Subir Halder
University of Limerick’s Dr Subir Halder discusses how device fingerprinting and machine learning could be used to detect cyberattacks in the growing world of smart manufacturing.
Before coming to the University of Limerick, Dr Subir Halder received a PhD in computer science and engineering from the Indian Institute of Engineering Science and Technology and was postdoctoral fellow at the University of Padua in Italy.
Now he is a Marie Sklodowska-Curie Actions fellow at Confirm, the Science Foundation Ireland research centre for smart manufacturing. From his base at the University of Limerick, he is developing approaches for the real-time detection of cyberattacks exploiting connected devices in smart manufacturing. He is also working on a platform to help manufacturing companies securely share data generated by connected devices with third-party services.
His research interests include security and privacy for wireless resource-constrained networks – in particular, designing security and privacy tech for smart environments, cloud computing, cyber-physical systems and autonomous vehicles.
‘Traditional security approaches that simply rely on building walls and defending the entry points will no longer suffice for securing smart factories’
– DR SUBIR HALDER
What inspired you to become a researcher?
I’ve always been enthusiastic to explore new opportunities and, as a source of inspiration, I always remember a famous quote from explorer Christopher Columbus: “You can never cross the ocean until you have the courage to lose sight of the shore.”
This instinct was my major drive to pursue research. In my work, I always try to find the answer to the question of how to ease human life by leveraging innovative technologies.
Can you tell us about the research you’re currently working on?
Smart manufacturing is expanding at an increasing pace due to the recent advances in information and communication technologies. While the number of connected industrial internet of things (IIoT) devices is increasing exponentially, complex and sophisticated cyberattacks continue to be a big challenge as connectivity of IIoT devices will significantly increase attack vectors for cyberattackers.
Currently, under the supervision of Dr Thomas Newe, I am investigating how to securely exchange and collaboratively analyse data across smart manufacturing supply chains while ensuring data integrity, accountability and traceability.
Particularly, I am developing a comprehensive cyber intelligence framework for smart manufacturing, which enables secure data exchange across the smart manufacturing ecosystem while providing a high degree of resilience, reliability, accountability and trustworthiness, and addressing cyberthreat detection and mitigation.
I plan to leverage two of the most promising cutting-edge technologies – device fingerprinting and machine learning – for detection of cyberattacks in smart manufacturing.
In your opinion, why is your research important?
Traditional security approaches that simply rely on building walls and defending the entry points will no longer suffice for securing smart factories. Instead, in my opinion, we need scalable, multi-layered and fully integrated strategic security approaches to protect manufacturing facilities against any cyberthreats.
We must detect cyberthreats and malware at an early stage across multiple layers ranging from the connected digital supply network via the smart factories down to the level of the connected IIoT devices themselves. Moreover, how to securely exchange and collaboratively analyse data across supply chains while ensuring data integrity, accountability and traceability – and hence realise the vision of collaborative manufacturing – are still challenges that have not been resolved.
What commercial applications do you foresee for your research?
Smart manufacturing will increase significantly in the near future with the adoption of the 5G connectivity and the deployment of fog and edge computing, which will further amplify the number of connected devices across the manufacturing sector.
However, with the current scenario, the vision of future intelligent and autonomous manufacturing units that exchange data and adapt to market changes is counterbalanced by an exponential increase in security risks and attack surfaces that emerge from the ever-growing connectivity of the production systems.
To overcome these challenges, I am designing an anomaly-detection technique leveraging a unique electronic fingerprint that each IIoT device creates via its physical characteristics to identify cyberattackers. Furthermore, due to rise in complex and sophisticated attacks, I am integrating machine learning techniques to automate the detection procedure and reduce the detection time.
Additionally, I am devising a secure data exchange platform by exploiting homomorphic encryption to protect sensitive data from unauthorised access by third-party services in smart manufacturing. I think all these works have significant commercial implication in the smart manufacturing domain.
What are some of the biggest challenges in your field?
Within digital supply networks, a key enabler driving the explosion of the digital collaboration ecosystem is a trusted data exchange. To address privacy concerns, but also empower manufacturing companies to exchange and share data, novel and practical solutions are needed. However, the existing solutions hardly ensure trusted data exchange among various actors in smart manufacturing ecosystems.
Additionally, IIoT adopts emerging technologies, exploits the information technology/operational technology convergence and offers new types of advanced manufacturing and industrial processes. As the number of connected devices and cloud networks increases within each organisation, the attack surface for data breaches or ransomware becomes greater and the need for innovative technologies comprising a wide range of fields becomes inevitable.
The existing approaches for detecting cyberattacks are typically based on either misuse detection or anomaly detection. In the context of the smart factory, the main drawback of misuse detection approaches is that they are unable to detect new, previously unseen attacks. Meanwhile, anomaly detection approaches often suffer from high false-alarm rates, limiting their applicability in practical settings.
Are there any common misconceptions about this area of research?
It is one of the biggest misconceptions that the industrial manufacturing domain is not particularly vulnerable to cyberattacks.
However, in a recent study by Kaspersky, it was revealed that security incidents and attacks targeting the manufacturing industry occupied third place in the most targeted industries, just after the financial services and information and communication technology industries.
What are some of the areas of research you’d like to see tackled in the years ahead?
The adaptation of numerous efficient machine learning techniques and deep learning approaches have transformed industrial automation significantly to deliver higher production efficiency.
However, with respect to cybersecurity, researchers have paid limited attention to design cognitive security mechanisms for situational awareness by exploiting machine learning techniques in smart manufacturing. Another aspect, in my opinion, that should be addressed in near future is the design of dynamic attack prevention approaches for smart manufacturing.
Don’t miss out on the knowledge you need to succeed. Sign up for the Daily Brief, Silicon Republic’s digest of need-to-know sci-tech news.