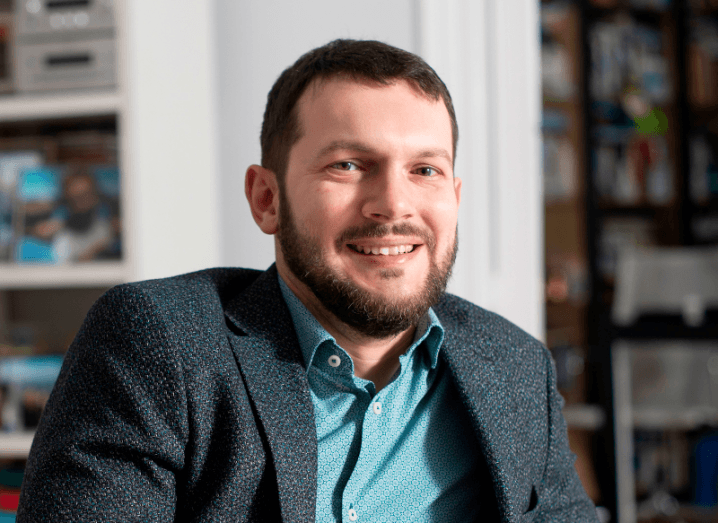
Victor Botev, Iris.ai. Image: Iris.ai
Iris.ai’s CTO and co-founder discusses the growth of the AI industry – particularly large language models – and how the tech will be adopted.
Victor Botev is the CTO and co-founder of Iris.ai, a Norwegian start-up that develops AI tools to assist academics, researchers and scientists during the research process.
The company was recently selected for a €2.4m grant and up to €12m in equity investments from the European Innovation Council Accelerator programme.
Before founding Iris.ai, Botev was a research engineer in artificial intelligence at Chalmers University of Technology.
In his current role, he heads up the research and product development for the company’s scientific text engine.
In an interview with SiliconRepublic.com, Botev said the features he’s directly involved in include the AI engine’s algorithms for text similarity, tabular data extraction, scientific text summarisation and domain-specific entity representation learning/disambiguation.
“The Iris.ai team’s tools are designed to help researchers better navigate the 2m research papers that are published every year. We enable researchers to rapidly pinpoint relevant literature and knowledge, dramatically speeding up the research and development processes,” he said.
‘System manipulation can be almost impossible to catch, and this is mainly down to a lack of explainability in AI’
– VICTOR BOTEV
What are some of the biggest challenges you’re facing in the current IT landscape?
Right now, we are riding the AI wave and witnessing generative AI take off. However, while large language models (LLMs) offer some substantial social and economic opportunities, they do come with challenges.
The biggest challenges we face with LLMs are the dubious results, as they can often fail on factual accuracy and knowledge validation. While the likes of Microsoft may have the technological and financial backing to tackle these issues with in-house capabilities, this won’t be a widely available solution.
To address this challenge, factually sensitive fields – which is everything related to science – will need to make use of small-scale models instead.
One example of this is Iris.ai’s platform. With many orders of magnitude fewer parameters than ChatGPT’s 175bn, our platform can generate more accurate results, as the model is built on high-quality data, not sheer volume.
This allows for easier domain adaptation, which in turn boosts the factuality of the results.
How can sustainability be addressed from an IT perspective?
AI is increasingly being absorbed into the day-to-day running of businesses and there is a definite place for this technology to create a more sustainable world.
Some sustainable AI applications include creating greener supply chains, improving energy grids and enforcing rigour around environmental monitoring and enforcement.
It is important, however, while exploring these fields that AI machines are as small and efficient as possible to reduce their environmental impact.
Furthermore, sustainability needs innovation. And innovation needs R&D. So, there is real value in leveraging AI in the research and development of greener products.
In the end, it’s the scientific community who are relied on by national and global policymakers to support sustainability and green ambitions. Therefore, greater integration of AI in R&D will be crucial to producing greener and more sustainable products and processes.
A use-case of AI in research and development is supported in building one of the largest databases and research banks of knowledge on material science.
Using this wealth of knowledge, the AI-powered search engine will guide the transition away from petrochemicals and steer toward creating sustainably sourced biomaterials.
What big tech trends do you believe are changing the world?
AI for broad use cases and an expansive user market have captured the headlines recently. This trend will begin to settle as more people confirm the open secret that some improvements must be made to allow AI tools to produce reliable outputs.
This, in turn, will prompt new techniques to increase trust and reliability in AI tools. However, until these are developed, we’ll see targeted applications for specific fields thrive.
By exploring these targeted solutions, we’ll see a widespread adoption of the technology, which will lead to innovative algorithms that will augment the models to optimise their size, memory consumption and use of data.
How can we address the security challenges currently facing the AI industry?
One of the most pressing security challenges for the AI industry is that infiltration can be very hard to spot.
System manipulation can be almost impossible to catch, and this is mainly down to a lack of explainability in AI.
While this is an issue now, as the technology becomes more widely utilised, the explainability will increase, helping to combat infiltration.
10 things you need to know direct to your inbox every weekday. Sign up for the Daily Brief, Silicon Republic’s digest of essential sci-tech news.