
Image: © Alex/Stock.adobe.com
Yashar Behzadi, CEO of Synthesis AI, explains what synthetic data is and how it could be used to drive automation and AI forward.
By 2022, there will be 45bn connected cameras in the world. Coupled with recent advances in deep learning, there is a tremendous opportunity to develop new and more capable AI-driven computer vision applications.
Up until now, computer vision has relied heavily on supervised learning in which humans label key attributes in an image. Attributes can include the type and location of objects in an image. The labelled data is then used to train computers to learn the relationship between input images and labels. However, this method has major disadvantages.
Companies are limited by the availability of sufficiently diverse and accurately human-labelled datasets. Currently, the time and cost to acquire and label image data is immense. A fundamental limitation of this approach is that a human worker can’t label all the attributes a company might be interested in.
This is especially important for technically complex areas of technology like autonomous vehicles, robotics, augmented reality and virtual reality. We simply can’t be limited by human labelling. Aside from the above limitations, real-world data presents a growing issue surrounding ethical use and privacy. The use of real-world data is only becoming more prohibitive as each country individually establishes compliance laws around data collection, data storage and more.
So what’s the answer? Synthetic data.
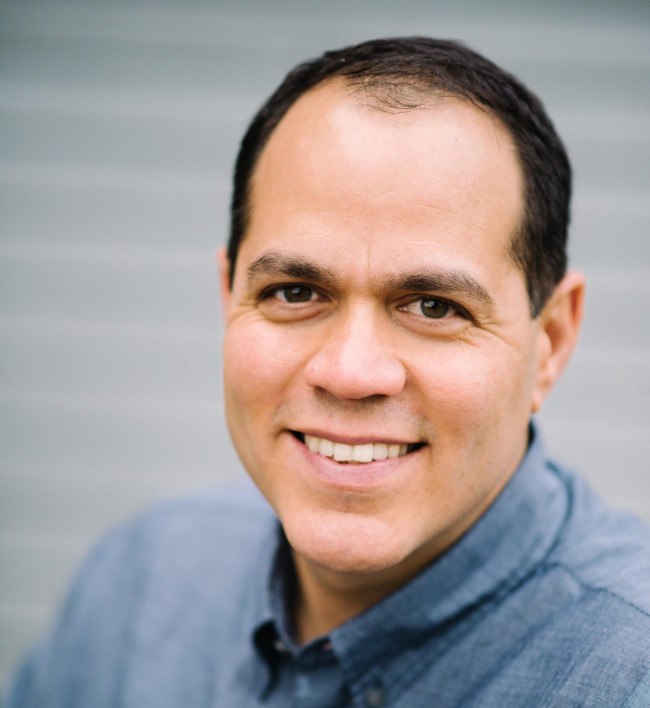
Yashar Behzadi. Image: Synthesis AI
Synthetic data is computer-generated image data that models the real world. Technologies from the visual effects industry are coupled with generative neural networks to create vast amounts of photo-realistic and automatically labelled image data. Synthetic data allows for the creation of training data at a fraction of the cost and time of current approaches.
In addition, since the data is generated, there are no underlying privacy concerns. Synthetic data enables the efficient prototyping, building and testing of complex computer vision systems.
Yet, more capable models are useless without applications that lead to return on investment. For many, the application and realisation of synthetic data is uncertain, so painting use cases is the most helpful for understanding its power.
To bring this new and unique technology to life, I’ve painted the top five use cases for synthetic data for the next 18 months:
Smart homes and assistants
The next generation of smart home and smart assistant products will include camera systems that will try to understand the behaviour of individuals within the context of their homes. The ability to identify objects, recognise human attention and emotions and understand interactions between people and the environment will be key.
Obtaining accurate labels of these complex environments is technically challenging and hindered by significant privacy concerns. With synthetic data, models can be built using highly diverse indoor virtual environments with simulated human models.
Smartphones
Our smartphones are packed with computer vision AI models. Facial verification models are used to ensure secure access to our phones and applications. Image segmentation and AI enhancement algorithms are used to process and optimise pictures.
As multi-lens and multi-modality cameras become ubiquitous in our phones, AI models will become even more sophisticated and begin to understand the 3D world around users.
Synthetic data’s enhanced labels (such as 3D positions and surface normals) will be a key enabler and are already in use by leading handset manufacturers to build powerful new capabilities.
Robotics
For robotics, the main value is speed in developing new capabilities. Currently, training a robot arm in real time requires a human worker to build the robotic arm or device, train it, test it, etc.
Leveraging synthetic data to simulate environments and robotic movements allows for the virtual training in machine time versus real time. Working in machine time with synthetic data will make robotic learning cheaper, faster and more scalable.
Additionally, new labels provided by synthetic data approaches related to 3D position, depth and new sensor systems will allow for the development of new and more capable models.
Autonomous vehicles
The fundamental value of synthetic data in autonomous vehicles, including drones, cars and more, is the introduction of rare events and the ability to test systems across a large set of scenarios.
A child running into the middle of the street is a rare event, as is a traffic light going out in a thunderstorm. Synthetic data enables autonomous vehicle manufacturers to easily add object detection to train computer vision models on how to handle such events efficiently and safely.
As new models are developed, testing against a bank of thousands of synthetically generated scenarios will also ensure well characterised and robust performance.
Teleconferencing
The future of work and meetings will be remote and empowered by new and improved teleconferencing capabilities. AI will enable better bandwidth utilisation, higher quality videos and enhanced features like emotion sensing and gesture recognition.
Synthetic data will play a key part in developing these systems as regulatory and privacy concerns limit the ability of companies to leverage consumer data.
Privacy concerns are even more important as these platforms become a key enabler of telemedicine. Building advanced features will also require highly accurate 3D labels that cannot be provided by traditional human-labelling approaches.
Synthetic data and its return on investment extends far beyond these five use cases and the good news is the future is bright. In the coming years, synthetic data will come to define a new paradigm in computer vision and enable the next generation of more capable models and products.
Yashar Behzadi is the CEO of Synthesis AI, a data generation platform using synthetic data technology to build more capable AI.