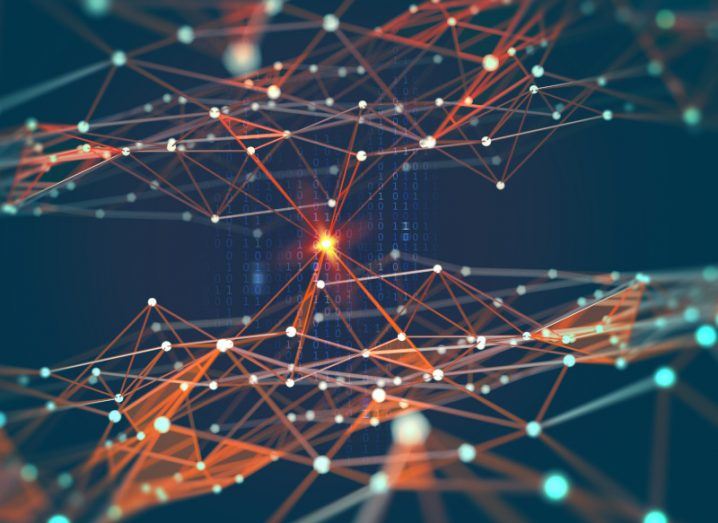
Image: © Siarhei/Stock.adobe.com
Huawei’s Alex Agapitos discusses how the latest data science techniques have become necessary in the maintenance of networks.
Data science has become essential in pretty much every industry that uses data, from supply chains and healthcare to insurance and e-commerce.
In the world of telecoms, data science techniques are required to optimise networks through predictive modelling techniques. To learn more about this, SiliconRepublic.com heard from Alex Agapitos, a principal AI architect at the Huawei Ireland Research Centre.
Agapitos has a degree in software engineering and a PhD in computer science. He worked as a post-doctoral researcher in the Complex and Adaptive Systems Laboratory at University College Dublin before joining Huawei in 2016.
He said the introduction of 5G, IoT and edge computing brings new complexities to network operations, which have made manual maintenance infeasible without the latest data science.
“Dominant success stories revolve around the use cases of reactive/predictive maintenance and network optimisation,” he said.
“In the former, outlier detection and predictive modelling techniques mine for patterns in historical data to accurately anticipate and warn about imminent network failures. This allows operators to identify early warning signs of failure and their associated root causes, enabling early interventions before failures affect end users.”
Agapitos said another important transformation that data science has brought is autonomous network optimisation.
“Deep learning-based predictive modelling allows simulation models of the network environment to be trained using historical data and then combined with data-driven optimisation algorithms that continuously reconfigure the network,” he said.
“The arrival of data-hungry applications including virtual reality, self-driving cars and gaming will further escalate the need for autonomous data-driven solutions in 5G and beyond.”
Data science trends in telecoms
With data science already driving autonomous network optimisation, Agapitos said he sees an era of “intelligent telecommunication networks” with “minimal human supervision” coming down the line.
“Advances in multi-agent systems will allow the network to be modelled and implemented as a collection of autonomous agents that perceive their environment and take actions to cooperatively meet a set of global goals, such us keeping the network performance at near-optimal levels at all times,” he said.
“To deal with ever-changing network conditions, it is crucial for autonomous agents to have the ability to continually acquire, fine-tune and transfer knowledge and skills throughout their life cycle, which is a research area known as continual or lifelong learning.”
Advancing lifelong learning for machine learning systems is an ongoing challenge but Agapitos said there is plenty of emerging research in this area.
He also said the advancing complexity and sophistication of intelligent telecommunication networks will inevitably pose a challenge to the human operator in understanding the reasoning behind autonomous decision-making.
“Trustworthiness of the autonomous system’s internal functionality is of fundamental importance and it will be realised through advances in explainable AI.”
Explainable AI is a research area that sits at the intersection of data science, deep learning and symbolic AI. The aim is to develop methods and techniques that produce accurate, explainable models of why and how an AI algorithm or prediction model arrives at a specific decision, so that the result can be understood by a human.
The question of privacy
While the need for data grows within society, so too does the question of privacy. Agapitos said he believes the issue of data privacy can be addressed via another area of data science – a machine learning technology known as federated learning.
“While standard machine learning approaches require centralising the training data in one machine or in the cloud, federated learning enables AI native network elements or user equipment to collaboratively learn a shared prediction model while keeping all the training data on-premise or on-device,” he said.
“In a nutshell, federated learning proceeds as follows: the network element or user equipment downloads the current model from a shared coordinator, it improves the model by online learning based on data generated locally at the network element or user equipment, and then summarises the model changes as a small model update.
“This small update is then sent back to the coordinator using encrypted communication, where it is immediately averaged with peer model updates to improve the shared model. Federated learning allows for smarter models, lower latency, less power consumption, all while ensuring privacy.”
Don’t miss out on the knowledge you need to succeed. Sign up for the Daily Brief, Silicon Republic’s digest of need-to-know sci-tech news.